When you’re an explorer on the Moon or Mars, time is in short supply. You’re running your checklists, checking your air reserves, making sure you don’t move too far away from the base. At the same time, you have some exploring to do! Your job – well, one of the many jobs you’ve been assigned – is to find out what the Moon, or Mars, is made of. And what better way to do this than to bring back some surface rocks.
But rocks look a lot alike in space. And you can’t bring 20 kg of rocks back to a lab ‘just in case’ – even if gravity is only a portion of what it is on Earth. Director of ESA’s CAVES and PANGAEA exploration programme Francesco Sauro stresses the importance of being efficient: “We cannot bring back a lot material from the Moon, so to be successful we need to bring back the most diverse material that we can get.”
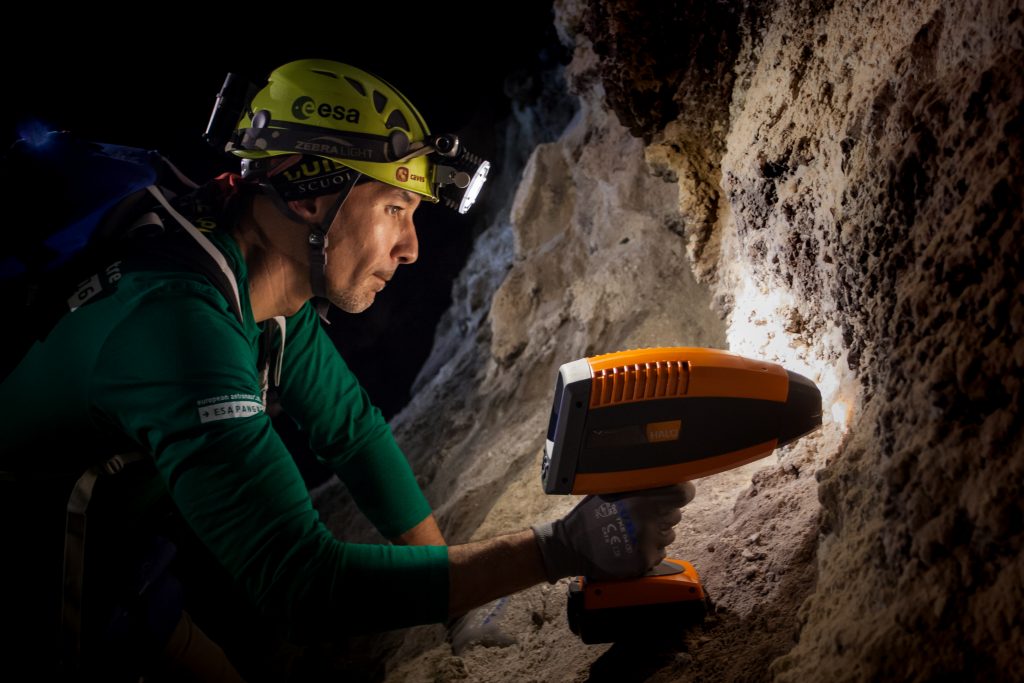
So, how do you do know which rocks you should take with you, and which ones would only take up valuable space?
Although experts can tell a lot by simply visually inspecting a rock, most samples are only truly identified using spectroscopy. This involves illuminating the sample and getting a unique ‘fingerprint’ reflected back at the spectrometer. These ‘spectral fingerprints’, or spectra can then be matched to those of known minerals. Previously, this had to be done in a lab, but now many spectrometers are small enough to take into the field.
The PANGAEA team built an extensive mineralogical database containing thousands of these fingerprints, including from minerals likely to be found on the Moon and on Mars. Spectroscopy data from the field can be matched to this library using an Electronic Field Book and subsequently identified.
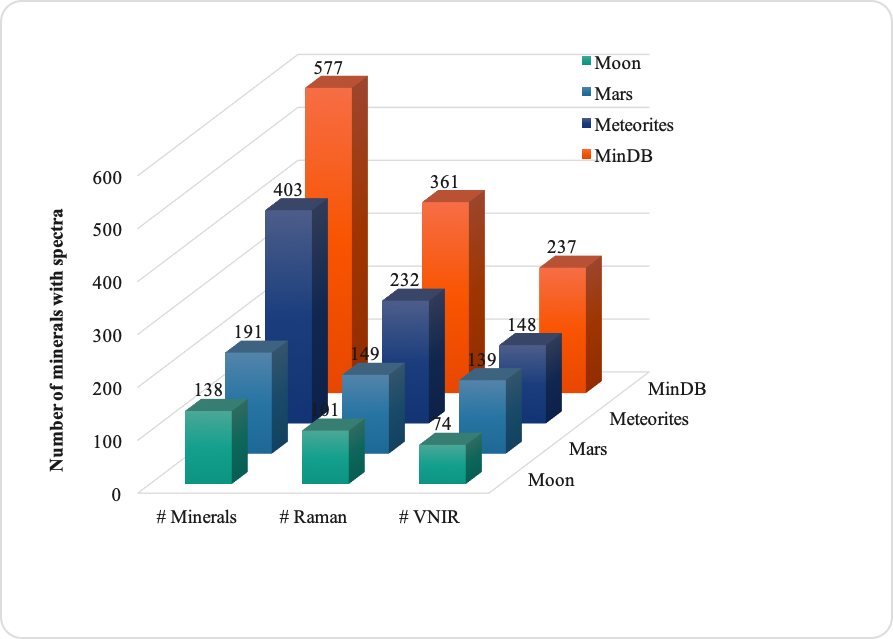
This works well in theory, but as always, real life is a bit more complicated. There are different spectrometers, each best suited for a certain type of mineral and less suitable for another. How do you decide which one you need if you don’t know which rock you have in front of you? This is where the PANGAEA team shines once more.
As explained in a recently published research paper, the team has added a new machine learning algorithm to the mineral database to greatly increase the speed and accuracy with which minerals can be identified.
The machine learning software combines input from complementary spectrometers, so that minerals can be identified more quickly and accurately. “We’ve used multi-spectral data fusion for the first time” explains Igor Drozdovskiy, Training Support Scientist at ESA, “and we’ve shown that using different types of spectroscopy data together greatly improves the accuracy.”
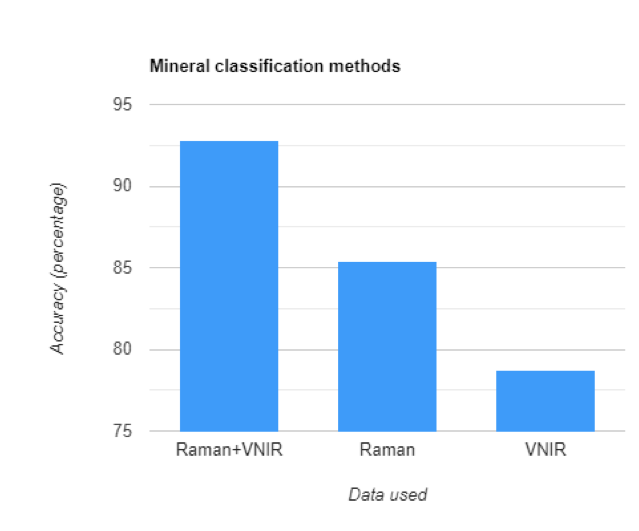
This is an important step towards creating a portable, decision-support tool for astronauts in the field. When combined with the right instrumentation, the software could provide an answer after just one minute of analysis, instead of the two or three days that lab analysis requires – when you’re on the Moon, this is crucial. Francesco is very happy: “The new software provides a base on which to build further. It’s very promising.”
The team is quick to stress that getting to this point was made possible by many people working together, proving once again that space exploration is a team sport. The list below this post further illustrates this.
And the good news is: work continues. Machine learning doesn’t stop. By simply running more queries and adding more spectra, the software automatically improves its accuracy.
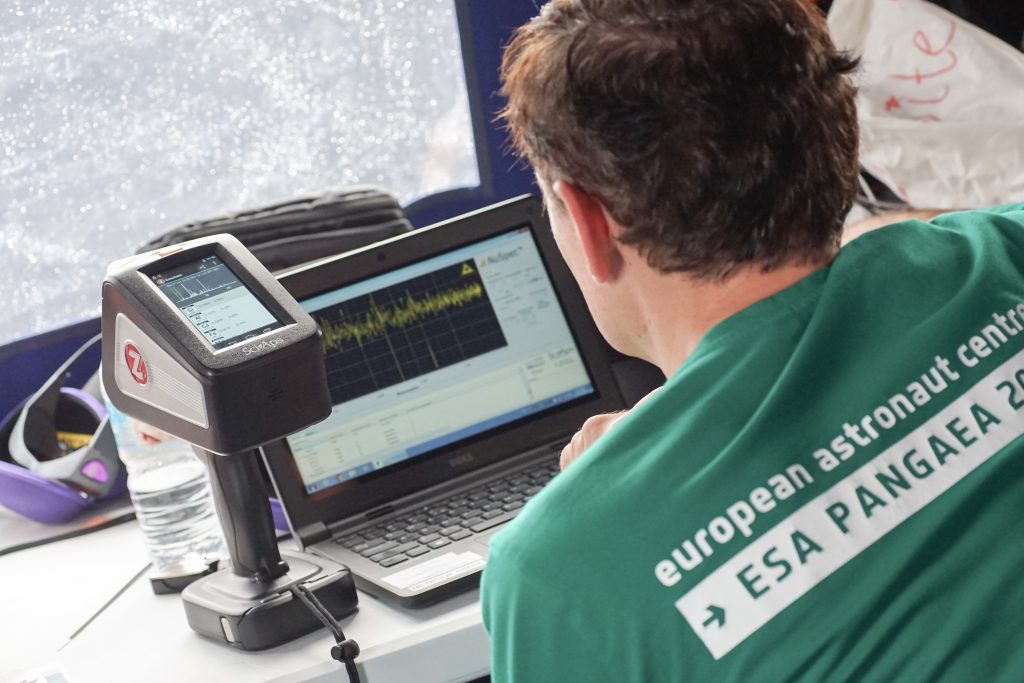
The PANGAEA team continues to look for ways to increase exploration efficiency. The ultimate goal is to have one tool that merges different spectroscopy methods in one instrument. Combined with the new identification software, it would give field geologists the support they need to autonomously make real-time decisions while exploring the Moon and Mars.
What is more, this research has a pleasant ‘side-effect’: the machine learning software can also provide much needed decision-support on Earth, in areas that you might not think of right away, such as the food industry, pharmaceutical development, medicine and conservation science to name a few. “Spectroscopy is everywhere” adds Igor “we look forward to sharing our research with other industries, improving real time decision-support not just for space exploration, but for many other Earth applications as well.”
PANGAEA internship students
- Machine Learning software for recognition of minerals
- Pavel Jahoda (Czech Technical University in Prague)
- Kristina Jarůšková (Czech Technical University in Prague)
- Mineralogical Database
- Gabriela Ligeza (ETH Zürich)
- Sam Cook (University of Bristol)
- Primož Vodnik (University of Ljubljana)
- Patrick Lennert (University of Erlangen-Nuremberg)
- Michael Franke (University Halle-Wittenberg)
- Analytical Tools
- Thelma Lestage (ISAE-ESTACA)
Collections and Spectroscopy of Planetary Analog Minerals
- Mineralogische Staatssammlung Munich / Mineralogical State Collection
- The Geopark of Lanzarote
- Geopark of Bletterbach
- The Riescrater Museum
- The Norwegian Mining Museum
- Mineralogical Museum Bonn
- GeoMuseum Cologne
- Institut für Planetologie, Münster
- Planetary Terrestrial Analog Library, https://www.ptal.eu/
Commercial Companies (developers and sellers in Europe of customized spectrometers)
University Collaborations
- University of Padova, Italy
- University of Bologna, Italy
- University of Münster, Germany
- University of Nantes, France
- University of Edinburgh, UK
- Instituto de Geociencias IGEO (CSIC-UCM), Spain
Discussion: no comments