These technologies aim to improve independent ways of working for humans and robots in space, especially during long-duration missions far from ground control teams on Earth. And Clara says, though it has been challenging, the remote nature of her internship helped provide greater insight into the way astronauts work in space.
An intelligent geological classifier
To save time and reduce the weight of samples astronauts return to Earth during future missions to the Moon, the Spaceship EAC team has been working on a machine learning algorithm. By automatically classifying lunar samples, it is designed to help astronauts decide which rocks are worth the journey and which to leave behind.
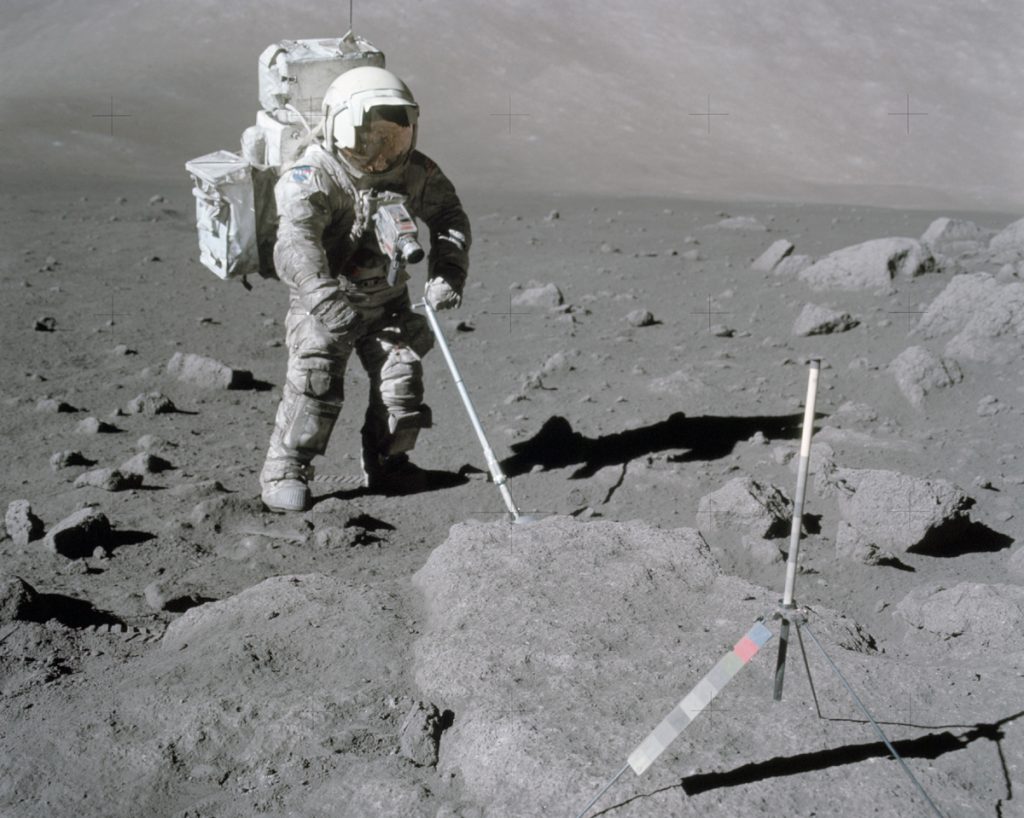
Apollo 17 rock sampling. NASA
For her project, Clara is using an unsupervised machine learning technique in which the model works on its own to discover patterns and information. This algorithm is particularly adept at processing and learning from image data, classifying rock fragments that have been cut into thin sections and analysed under a polarised light microscope. The more images the algorithm is provided with, the more it learns from the different image information.
“We are creating a model that learns from a database. Then, if the model is good enough, it can be applied to new data (future lunar samples) that it has never seen before, and the model will be able to identify the rocks and their compositions for the astronauts without requiring them to have advanced geological knowledge” Clara explains.
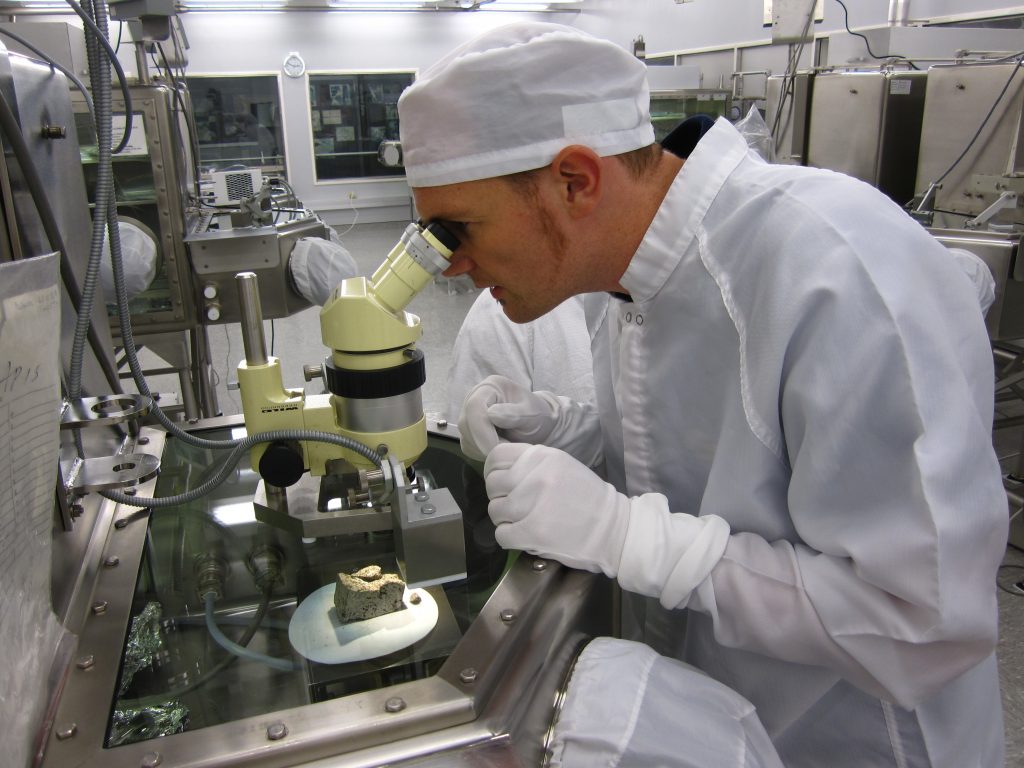
ESA astronaut Alexander Gerst examines Apollo lunar rocks at NASA’s Lunar Sample Laboratory. ESA-A. Gerst
Based on a geological classifier developed by former Spaceship EAC intern Piotr Knapczyk, Clara’s goal is now to expand the database by developing clustering methods that help identify different mineral types on the thin section images of lunar rock samples. “Once we have identified the clusters of minerals located on the image, we can name them and learn more about the composition of lunar rocks,” she says.
Thin Section Photograph of Apollo 11 Sample 10003,153 in Plane-Polarized Light (left) and in Cross-Polarized Light (right). NASA
Thin section image in Plane-Polarized Light (left) and in Cross-Polarized Light (right) clustered by the algorithm. All pixels of the same colour correspond to the same mineral. ESA-C. Moriceau
One big challenge Clara sees with machine learning methods like this is the completeness of data available. “When I am working on the current dataset about lunar rock samples, for example on the mineral clusters, I have to supplement it with data from other sources at the moment,” she explains.
Today this method is still being tested and trained on Earth using datasets from the Apollo missions. To make sure of this classification algorithm during future space missions, astronauts or planetary rovers will need to be equipped with special microscopes and produce the thin-section images required.
An expert system for space photography
Clara’s second project aims at facilitating space photography for astronauts. On board the International Space Station, astronauts take pictures of Earth and space from the Cupola, the observatory of the space station. But as the Space Station is moving, they only have a few seconds to adjust the camera settings and angle to achieve that perfect shot.
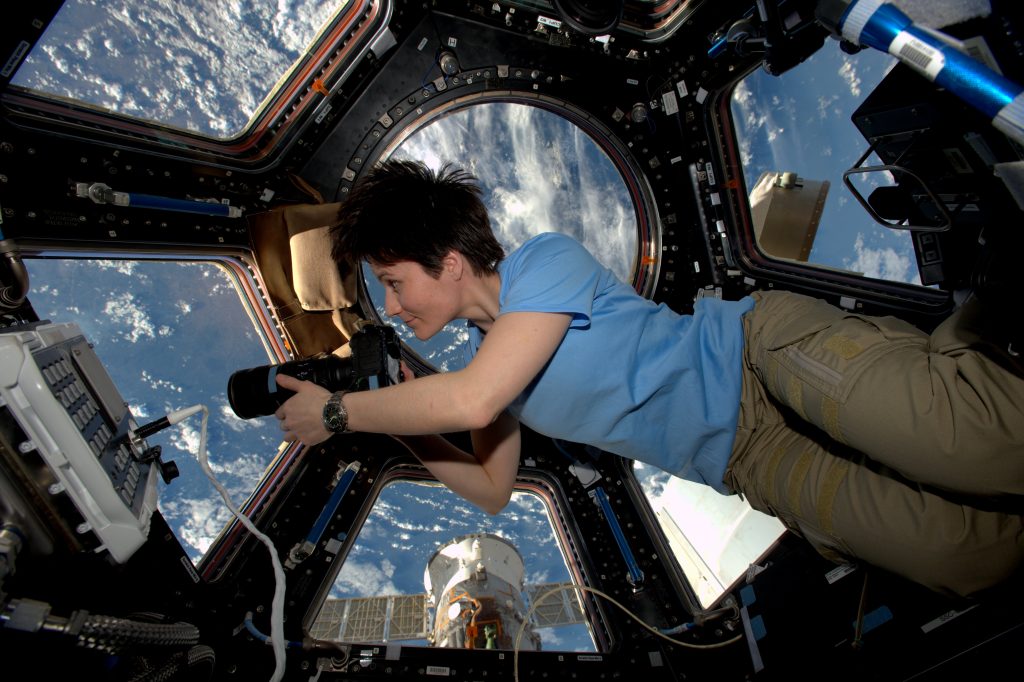
ESA astronaut Samantha Cristoforetti in the cupola of the ISS. ESA/NASA
To help this, Clara is working on a decision-making algorithm designed to observe external environmental conditions, like weather or light, in order to provide the astronauts with camera recommendations before they take their photo.
“This is a bit tricky as I am not a specialist in space photography,” Clara explains. “But most astronauts need to be trained before their mission to take good pictures in space, so I am lucky to be surrounded by experts and astronauts who are more than happy to share their experience and help me with this project,” she adds.
Looking ahead
Different AI systems, like the ones Clara is working on, can complement each other and will become more important in the future.
“In the long-term, I think developing machine learning algorithms as well as human-robot-collaboration is key for future space exploration missions, especially when we go further to the Moon and Mars and cannot depend on permanent or immediate ground support anymore,” she says.
Due to Covid-19 restrictions, Clara has to work from home throughout her internship. As with the scenarios for her projects in isolated space environments, Clara says that working remotely is more challenging and means you have to adapt and communicate in a different way. Yet, she is very happy about the continuous support and cooperation she experienced in the team.
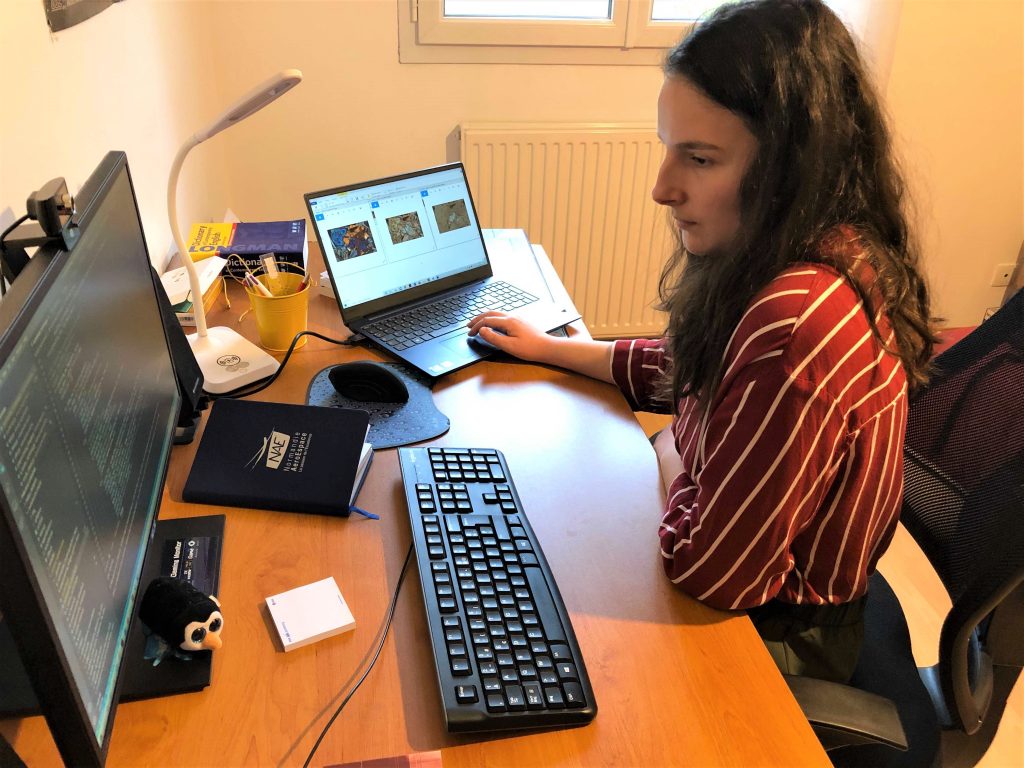
Clara working remotely on her projects. ESA-C. Moriceau
“I am amazed to see how the Spaceship EAC team adapted to the situation and made me feel part of the team from my very first day. Even remotely, I have the opportunity to exchange with many other students and experts from EAC which is very rewarding. What I love the most is that I am learning something new every day!”
Learn more about the Spaceship EAC programme here
Meet more students and Spaceship EAC interns here